You Can Score Higher
Discover the power of Embibe’s MEDHAS platform, the next level of learning and assessment technology! Our cutting-edge platform leverages the latest natural language understanding (NLU) technology advancements to provide hyper-tagged learning and assessment content, contextualised knowledge graphs, and interpretable predictions that enable personalised and adaptive learning experiences.
Medhas, a Sanskrit word, means knowledge, understanding and intelligence. Natural language understanding (NLU) platform is very important to build an EdTech AI platform. NLU capabilities enable hyper-tagged learning and assessment content, contextualized knowledge graphs, and interpretable and explainable predictions to enrich, recommend or create content for students who need to achieve learning outcomes. A few examples where it directly helps achieve state-of-the-art performance are content intelligence tasks like question generation, question answering, doubt resolution, insta-solver, etc.
Artificial Intelligence has come a long way, with deep learning techniques making a solid case for its potential over a wide range of domain applications. However, the current state of artificial intelligence is dominated by black-box models trained by learning latent patterns statistically captured in training data. These models are inexplicable and potentially vulnerable to explicitly defined domain scenarios.
Platform Medhas aims to:
- Make deep learning models explainable and interpretable
- Infuse domain knowledge explicitly defined in Knowledge Graphs into deep learning models
- Make deep learning models accessible on edge devices and resource-constrained settings.
- In other words, model optimization to make the local on-device inference.
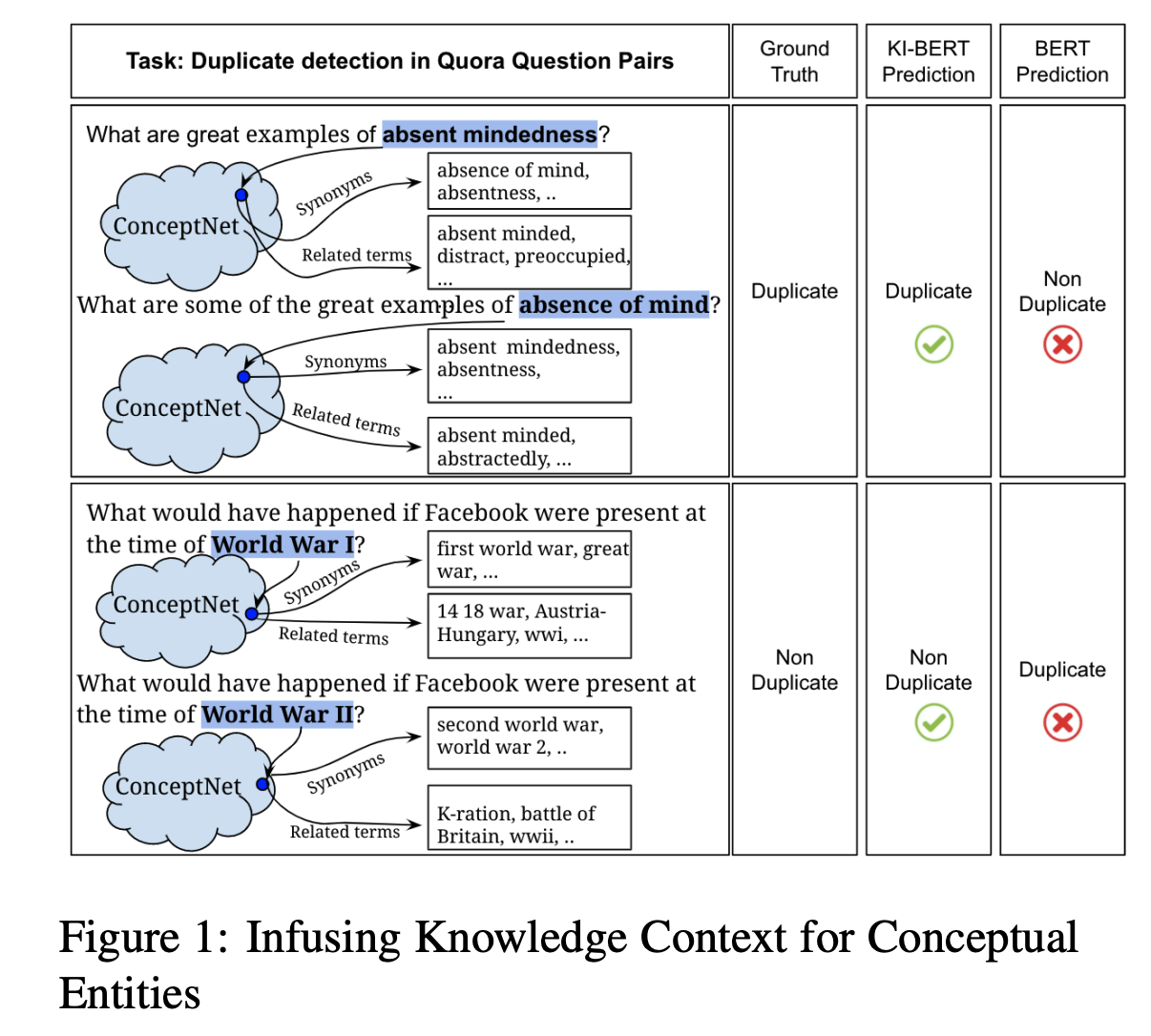
We infuse knowledge embedding from knowledge graphs into self-attention-based models. For example, we took the embedding of conceptual entities like “absent-mindedness” from ConceptNet and infuse it with token embeddings of the same into BERT. The same process of infusing knowledge embedding for “absence of mind”. So, the next self-attention layer will pay attention to the combined knowledge and token embeddings of “absent-mindedness” and “absence of mind” entities.
As knowledge embedding of “absent-mindedness” and “absence of mind” will be very similar, the model will get the idea that these two are similar entities.
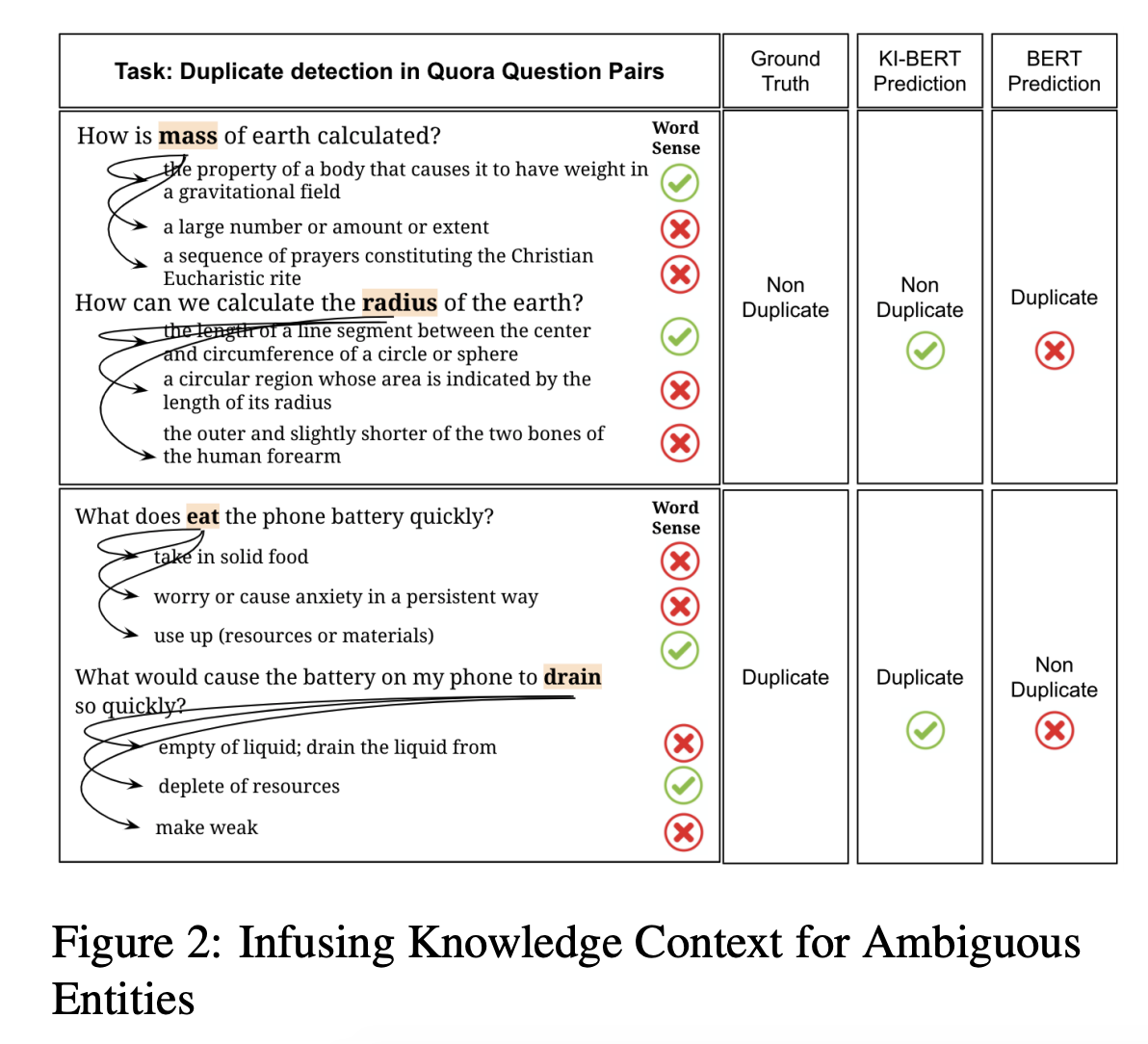
In the above example, we fetch knowledge embedding of ambiguous entities like “mass” and “radius” from WordNet and infuse it with token embeddings of the same into the self-attention layer of BERT.
So, the next self-attention layer will pay attention to combined knowledge embedding and token embedding of the ambiguous entities, which will help the model understand the input sentence better.
How infusing knowledge gives more interpretable confidence:
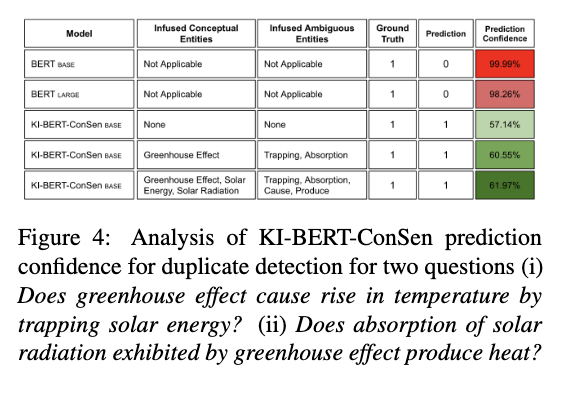
We can see in the above example(second last row) that, after adding Conceptual entities like “Greenhouse Effect” and ambiguous entities like “Trapping” and “Absorption”, not only did it make the correct prediction, but the confidence of making the prediction also increased to 60.55% as we added more entities.
When we also added more ambiguous and conceptual entities like, “Solar Energy” and “Cause”, respectively, confidence increased even more to 61.97%.
To summarise, as we add more meaningful entities, not only it made correct predictions(unlike vanilla BERT was making wrong predictions), but it also increased the confidence of the model.
Platform Medhas is built by the Education Research Lab at Embibe. Education Research Lab is a niche disciplined effort to identify key open problems with colossal impact and push state-of-the-art research by inventing original value propositions.
Reference:
[1] Gaur, Manas, Keyur Faldu, and Amit Sheth. “Semantics of the Black-Box: Can knowledge graphs help make deep learning systems more interpretable and explainable?.” IEEE Internet Computing 25, no. 1 (2021): 51-59.
[2] Faldu, Keyur, Amit Sheth, Prashant Kikani, and Hemang Akabari. “KI-BERT: Infusing Knowledge Context for Better Language and Domain Understanding.” arXiv preprint arXiv:2104.08145 (2021).
[3] Gaur, Manas, Ankit Desai, Keyur Faldu, and Amit Sheth. “Explainable AI Using Knowledge Graphs.” In ACM CoDS-COMAD Conference. 2020.
[4] Keyur Faldu. “Rise of Modern NLP and the Need of Interpretability!” Towards Data Science, August 2020.
[5] Keyur Faldu, Dr Amit Sheth. “Discovering the Encoded Linguistic Knowledge in NLP models.” Towards Data Science, September 2020.
[6] Keyur Faldu, Dr Amit Sheth. “Linguistics Wisdom of NLP Models.” Towards Data Science, November 2020.
[8] Faldu, Keyur, Aditi Avasthi, and Achint Thomas. “Adaptive learning machine for score improvement and parts thereof.” U.S. Patent 10,854,099, issued December 1, 2020.
[9] Dhavala, Soma, Chirag Bhatia, Joy Bose, Keyur Faldu, and Aditi Avasthi. “Auto Generation of Diagnostic Assessments and Their Quality Evaluation.” International Educational Data Mining Society (2020).
[10] Faldu, Keyur, Achint Thomas, and Aditi Avasthi. “System and method for recommending personalized content using contextualized knowledge base.” U.S. Patent Application 16/586,512, filed October 1, 2020.
[11] Thomas, Achint, Keyur Faldu, and Aditi Avasthi. “System and method for personalized retrieval of academic content in a hierarchical manner.” U.S. Patent Application 16/740,223, filed October 1, 2020.
[12] “#RAISE2020 – Embibe – AI-Powered learning outcomes platform for personalized education”, MyGov India, Oct 2020, https://www.youtube.com/watch?v=kuwFtHgN3qU
← Back to AI home